Control & Optimization
The development of control and optimization methods for dynamical systems is the natural research focus of the Chair of Automatic Control. In particular, we focus on nonlinear and predictive control concepts as well as path/trajectory planning for dynamical systems closely related to optimization-based methods, always having an eye on the real-time and embedded realization for practical applications.
Research on nonlinear systems and control is at the heart of the Chair of Automatic Control. Modern control concepts such as model predictive control (MPC) often rely on optimization problems that have to be solved online. In particular mechatronic systems often require sampling times in the (sub-)millisecond range and therefore highly efficient control algorithms and warm-start strategies. The Chair of Automatic Control has long standing experience with the modeling of control problems of different physical domains and the development of nonlinear and predictive control concepts, always with the intention to bring these methods into practice. We also develop and maintain the open source MPC toolbox GRAMPC that was successfully used in many research and industrial projects and by other research groups. Current research concerns the extension to stochastic nonlinear systems to account for uncertainties in a consistent probabilistic setting.
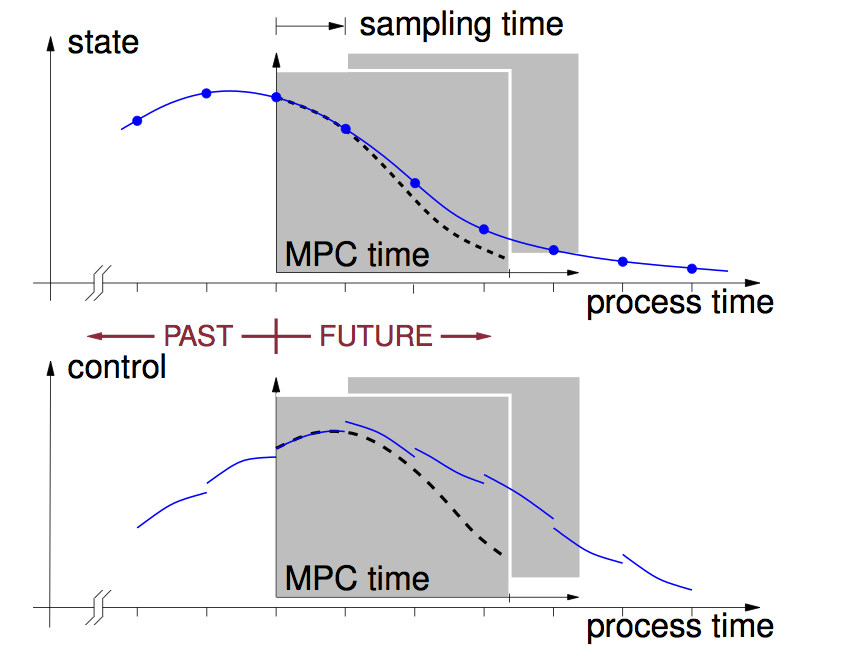
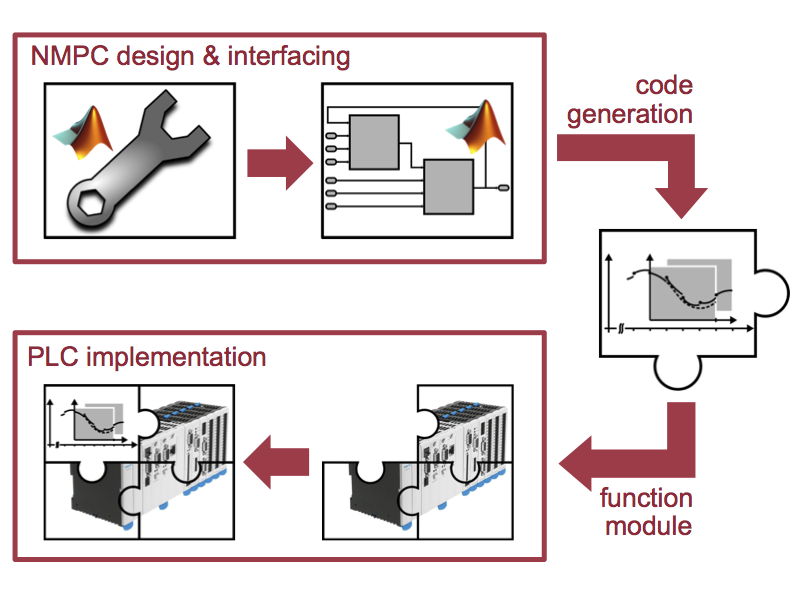
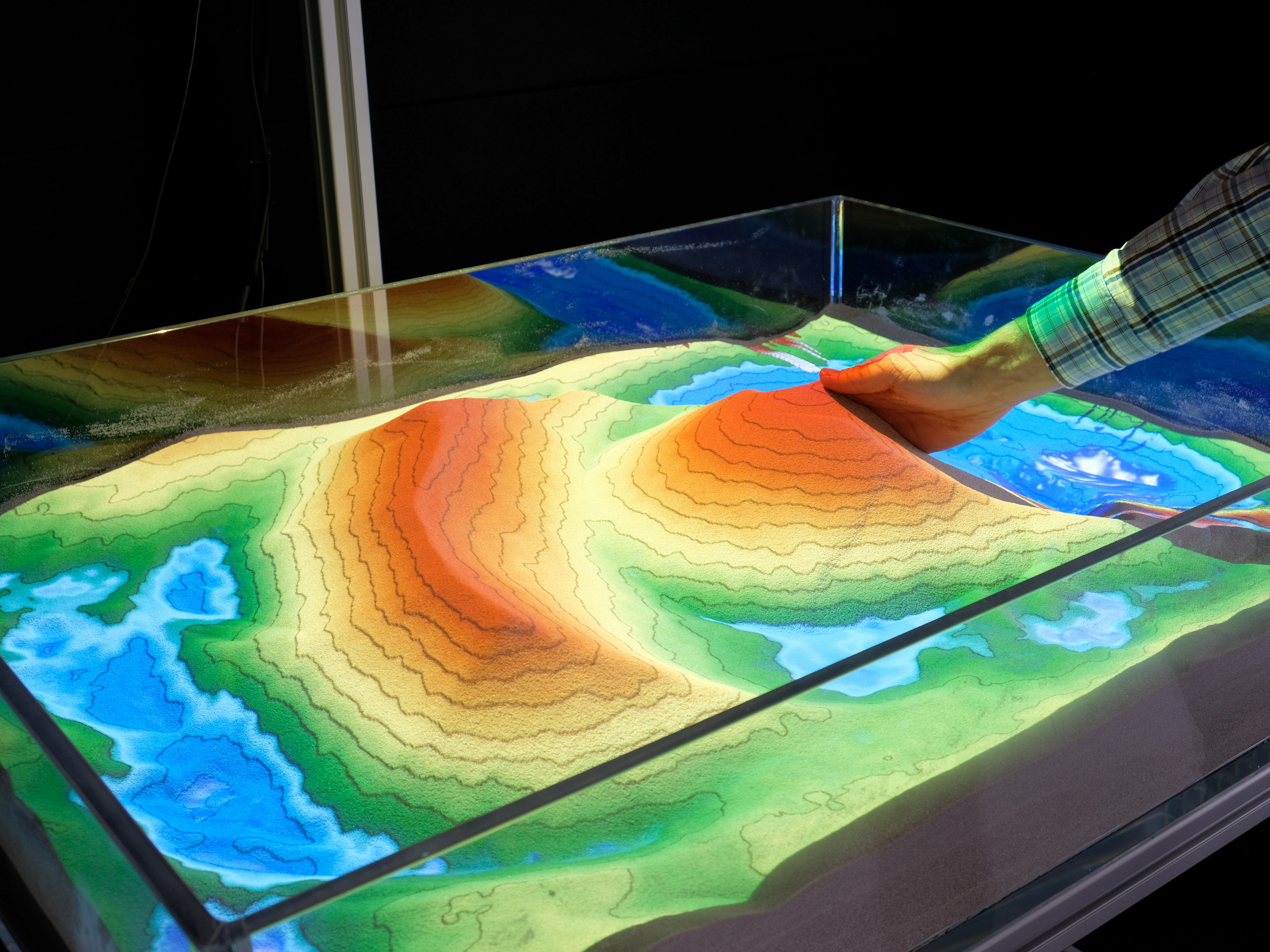
Beyond the “classical” centralized view on control applications, networked systems are of increasing importance, not only in terms of autonomous and mobile robots, distributed energy networks (smart grids), but also in connection with industry 4.0 and flexible production. The control of networked systems is challenging, because centralized approaches do not scale well with the number of subsystems and do not provide the flexibility for plug-and-play or reconfiguration scenarios. We focus on both the design of distributed (model predictive) control schemes for networked systems as well as the agent-based distributed implementation of these concepts along with suitable communication concepts to enhance the overall efficiency of networked systems. An outcome of this research is the open-source framework GRAMPC-D that implements a real-time efficient ADMM algorithm for distributed model predictive control of nonlinear networked systems including plug-and-play functionality.
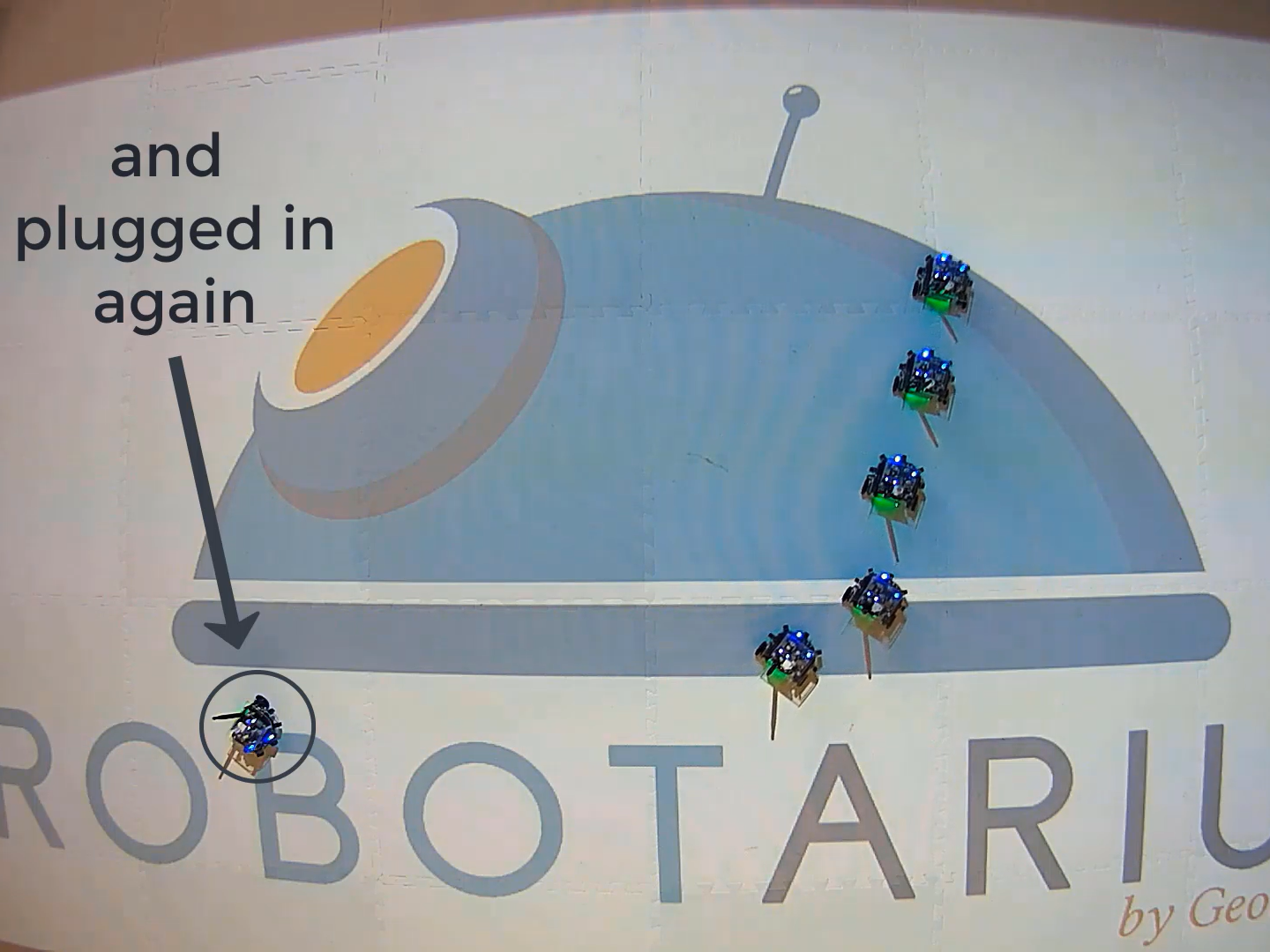
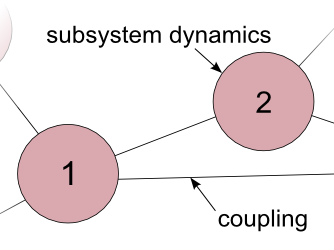
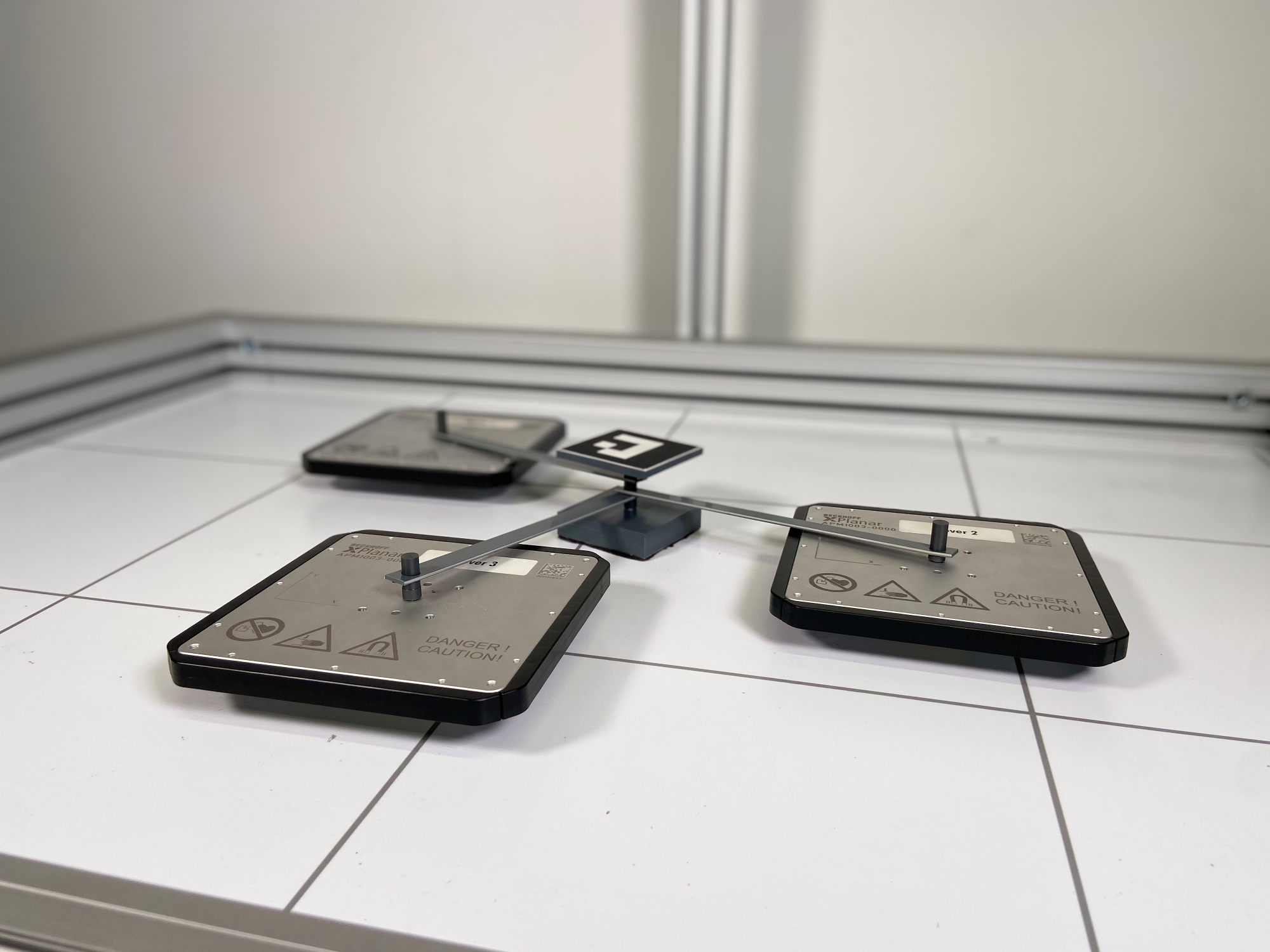
Videos
Model predictive control
Related projects
Feasibility of stochastic model predictive control for autonomous driving
SPP 2364: Formulation of dispersed systems via (melt) emulsification: Process design, in situ diagnostics and regulation
The aim of this project is the automated production of liquid-liquid disperse systems via melt emulsification, whereby in this process emulsification takes place at elevated temperature. The products obtained after cooling are dispersions of spherical nanoparticles or microparticles. Within the scope of this project, a melt emulsification device for the automated production of product particles with a well-defined particle size distribution (PSD) will be further developed. The PSD has a significant influence on the subsequent product properties, such as flow behavior or drug release kinetics. The PSD of the products is determined by the complex interaction of competing mechanisms. These are, in particular, droplet breakup in a rotor-stator device as a result of shear and elongation stress, as well as coalescence and further ripening, which in turn depend on the system composition, i.e. the emulsifier used (type, concentration) and the dispersion phase (viscosity, volume fraction).
Therefore, for a better process understanding and an active process control, possibilities for in situ determination of the PSD are urgently required. In this project, a novel fiber-coupled measurement system based on broadband elastic light scattering is developed for in situ measurement of the PSD. The system will be validated on reference particle systems and applied to the emulsification process. Furthermore, a hybrid process model is developed, which is the basis for the design of a model predictive control of the process. The model predictive control in combination with the in situ measurement will provide the possibility for an active process control and the production of emulsions with predefined properties and a simultaneous optimization of the process time.
Control of ring resonator modulators in optical communication
Cooperative manipulation with dual-arm robots at the payload limit
Dual-armrobots offer a high potential for automation technology, as they canbe used to implement tasks that are not possible with one arm alone.This includes in particular the manipulation of large or heavyobjects that exceed the payload of a single arm. Illustrativeexamples are the movement of beverage crates, long boards or pipes,which are also preferably grasped by humans with both hands.
However,cooperative manipulation is particularly challenging, because botharms…
Anomaly detection and intelligent recalibration of sensorized systems
Distributed model predictive control of nonlinear systems with asynchronous communication
KI-unterstützte Modellierung zur Steigerung der Regelgüte
Robust control of modular multi-level converters
Innovative Regelungs- und Steuerungsstrategien für Druckerhöhungsanlagen - TP Erlangen
Motion planning for driving simulators
Automated path planning for truck-trailer configurations
AGENT: Agent-based systems for intelligent and robust control of complex energy systems in non-residential buildings as part of a superordinate energy system
The consortium of the project AGENT consists of the partners RWTH Aachen, Friedrich-Alexander- Universität Erlangen-Nürnberg and Robert Bosch GmbH, who already conduct research in the field of building energy system technology and building automation technology. The practitioners’ perspective shows that increasing complexity of energy systems within non-residential buildings leads to further challenges within their operation. These are caused by complex interaction and the attempt to control such systems by means of a central instance using a supervisory control system. The goal in this project is the development of a future building automation system based on agents. A building with its energy system will be enabled to optimize not only its own operation but to serve as a part of a superordinate energy system and to behave as a grid-friendly building. The agent-based system will be self-configuring and, for instance, optimize the energy consumption of the building. Therewith, it reduces the effort of construction, commissioning and operation of energy and building energy systems. For the task of controlling energy systems, generic tasks of single components and groups of components will be defined and included into a practitioner’s guide. Practical usability will be ensured to allow for dissemination in the building energy sector.
Modular distributed model predictive control of nonlinear systems with neighborhood models
The steadily growing demands on efficiency and flexibility of modern automation and control systems requires a broader design approach for the overall system that goes beyond the isolated look at and control of single subsystems. Decentral and distributed control schemes follow this holistic design approach by including the interdependencies between the subsystems in the control design.
Model predictive control (MPC) appears to be a suitable control approach to tackle these kind of systems. In essence, MPC relies on the numerical solution of a finite-horizon dynamic optimization problem that is repetitively solved according to the sampling rate of the system. An extension of MPC to coupled systems is distributed MPC (DMPC), which assigns a single communicating MPC agent to each subsystem.
The goal of the project is to develop a DMPC scheme for nonlinear coupled systems, where each MPC agent contains a neighborhood model that anticipates the dynamical behavior of its neighbors in order to enhance the convergence and robustness of the distributed algorithm. Besides the development and mathematical investigation of the methodology, a further goal of the project is the numerical and experimental realization of the control approach. A particular intention of the project is to develop a modular framework that allows for an easy configuration and adaptation of the coupling structure for suitable system classes.
Trajectory planning for off-road applications
Compliance for a robotic assistance system
Regelung des Antriebsstrangs beim Kaltwalzen
Robust energy-based control of MMC/HVDV systems
Model predictive flight control
Related publications
Since 2022
- Harder, K., Niemeyer, J., Remele, J., & Graichen, K. (2023). Hierarchical model predictive control for an off-highway Diesel engine with SCR catalyst. International Journal of Engine Research. https://doi.org/10.1177/14680874221143600
- Landgraf, D., Völz, A., Kontes, G., Graichen, K., & Mutschler, C. (2022). Hierarchical learning for model predictive collision avoidance. In IFAC PapersOnLine (pp. 355-360). Vienna (Austria).
- Landgraf, D., Völz, A., & Graichen, K. (2022). Nonlinear model predictive control with latent force models. In Proceedings 2022 American Control Conference (ACC) (pp. 4979-4984). Atlanta, GA (USA).
- Snobar, F., Reinhard, J., Huber, H., Hoffmann, M., Stelzig, M., Vossiek, M., & Graichen, K. (2022). FOV-based model predictive object tracking for quadcopters. In Proceedings of the 9th IFAC Symposium on Mechatronic Systems (Mechatronics 2022) (pp. 13 - 18). Los Angeles, CA (USA).
- Stecher, J., Kiltz, L., & Graichen, K. (2022). Semi-infinite programming using Gaussian process regression for robust design optimization. In Proceedings European Control Conference (pp. 52-59). London (UK).
- Harder, K., Niemeyer, J., Remele, J., & Graichen, K. (2023). Hierarchical model predictive control for an off-highway Diesel engine with SCR catalyst. International Journal of Engine Research. https://doi.org/10.1177/14680874221143600
- Landgraf, D., Völz, A., Kontes, G., Graichen, K., & Mutschler, C. (2022). Hierarchical learning for model predictive collision avoidance. In IFAC PapersOnLine (pp. 355-360). Vienna (Austria).
- Landgraf, D., Völz, A., & Graichen, K. (2022). Nonlinear model predictive control with latent force models. In Proceedings 2022 American Control Conference (ACC) (pp. 4979-4984). Atlanta, GA (USA).
- Snobar, F., Reinhard, J., Huber, H., Hoffmann, M., Stelzig, M., Vossiek, M., & Graichen, K. (2022). FOV-based model predictive object tracking for quadcopters. In Proceedings of the 9th IFAC Symposium on Mechatronic Systems (Mechatronics 2022) (pp. 13 - 18). Los Angeles, CA (USA).
- Stecher, J., Kiltz, L., & Graichen, K. (2022). Semi-infinite programming using Gaussian process regression for robust design optimization. In Proceedings European Control Conference (pp. 52-59). London (UK).
2021
- Völz, A., & Graichen, K. (2021). Gradient-based nonlinear model predictive control for systems with state-dependent mass matrix. In Proceedings of the 2021 IEEE Conference on Decision and Control (CDC), accepted.
- Völz, A., & Graichen, K. (2021). Gradient-based nonlinear model predictive control for systems with state-dependent mass matrix. In Proceedings of the 2021 IEEE Conference on Decision and Control (CDC), accepted.
2020
- Englert, T., & Graichen, K. (2020). Nonlinear model predictive torque control and setpoint computation of induction machines for high performance applications. Control Engineering Practice, 99. https://doi.org/10.1016/j.conengprac.2020.104415
- Lomakin, A., Mayr, A., Graichen, K., & Franke, J. (2020). Optimization of direct winding processes based on a holistic control approach. In Proceedings of the Electric Drives Production Conference (E-DPC). Ludwigsburg (D).
- Kruse, T., & Graichen, K. (2020). Moving horizon estimation for continuous glucose monitoring. In Proceedings of the 7th International Conference on Biomedical Engineering and Systems (ICBES 20).
- Englert, T., & Graichen, K. (2020). Nonlinear model predictive torque control and setpoint computation of induction machines for high performance applications. Control Engineering Practice, 99. https://doi.org/10.1016/j.conengprac.2020.104415
- Lomakin, A., Mayr, A., Graichen, K., & Franke, J. (2020). Optimization of direct winding processes based on a holistic control approach. In Proceedings of the Electric Drives Production Conference (E-DPC). Ludwigsburg (D).
- Kruse, T., & Graichen, K. (2020). Moving horizon estimation for continuous glucose monitoring. In Proceedings of the 7th International Conference on Biomedical Engineering and Systems (ICBES 20).
2019
- Bestler, A., & Graichen, K. (2019). Distributed model predictive control for continuous-time nonlinear systems based on suboptimal ADMM. Optimal Control Applications & Methods, 40(1), 1-23. https://doi.org/10.1002/oca.2459
- Bestler, A., & Graichen, K. (2019). Distributed model predictive control for continuous-time nonlinear systems based on suboptimal ADMM. Optimal Control Applications & Methods, 40(1), 1-23. https://doi.org/10.1002/oca.2459
2018 and earlier
- Rhein, S., Utz, T., & Graichen, K. (2014). Efficient state constraint handling for MPC of the heat equation. In Proceedings 2014 UKACC 10th International Conference on Control (pp. 663-668). Loughborough (UK).
- Käpernick, B., Hentzelt, S., & Graichen, K. (2013). A parallelizable decomposition approach for constrained optimal control problems. In Proceedings 52th IEEE Conference on Decision and Control (CDC) (pp. 5783-5788). Florence (Italy).
- Rhein, S., & Graichen, K. (2018). Constrained trajectory planning and actuator design for electromagnetic heating systems. Control Engineering Practice, 74, 191-203.
- Käpernick, B., Süß, S., Schubert, E., & Graichen, K. (2014). A synthesis strategy for nonlinear model predictive controller on FPGA. In Proceedings 2014 UKACC 10th International Conference on Control (pp. 662-667). Loughborough (UK).
- Graichen, K., & Käpernick, B. (2012). A real-time gradient method for nonlinear model predictive control. In Zheng T (Eds.), Frontiers of Model Predictive Control. (pp. 9-28). InTech (open access).
- Rhein, S., Utz, T., & Graichen, K. (2014). Efficient state constraint handling for MPC of the heat equation. In Proceedings 2014 UKACC 10th International Conference on Control (pp. 663-668). Loughborough (UK).
- Käpernick, B., Hentzelt, S., & Graichen, K. (2013). A parallelizable decomposition approach for constrained optimal control problems. In Proceedings 52th IEEE Conference on Decision and Control (CDC) (pp. 5783-5788). Florence (Italy).
- Rhein, S., & Graichen, K. (2018). Constrained trajectory planning and actuator design for electromagnetic heating systems. Control Engineering Practice, 74, 191-203.
- Käpernick, B., Süß, S., Schubert, E., & Graichen, K. (2014). A synthesis strategy for nonlinear model predictive controller on FPGA. In Proceedings 2014 UKACC 10th International Conference on Control (pp. 662-667). Loughborough (UK).
- Graichen, K., & Käpernick, B. (2012). A real-time gradient method for nonlinear model predictive control. In Zheng T (Eds.), Frontiers of Model Predictive Control. (pp. 9-28). InTech (open access).